AI with Retrieval Augmented Generation (RAG)
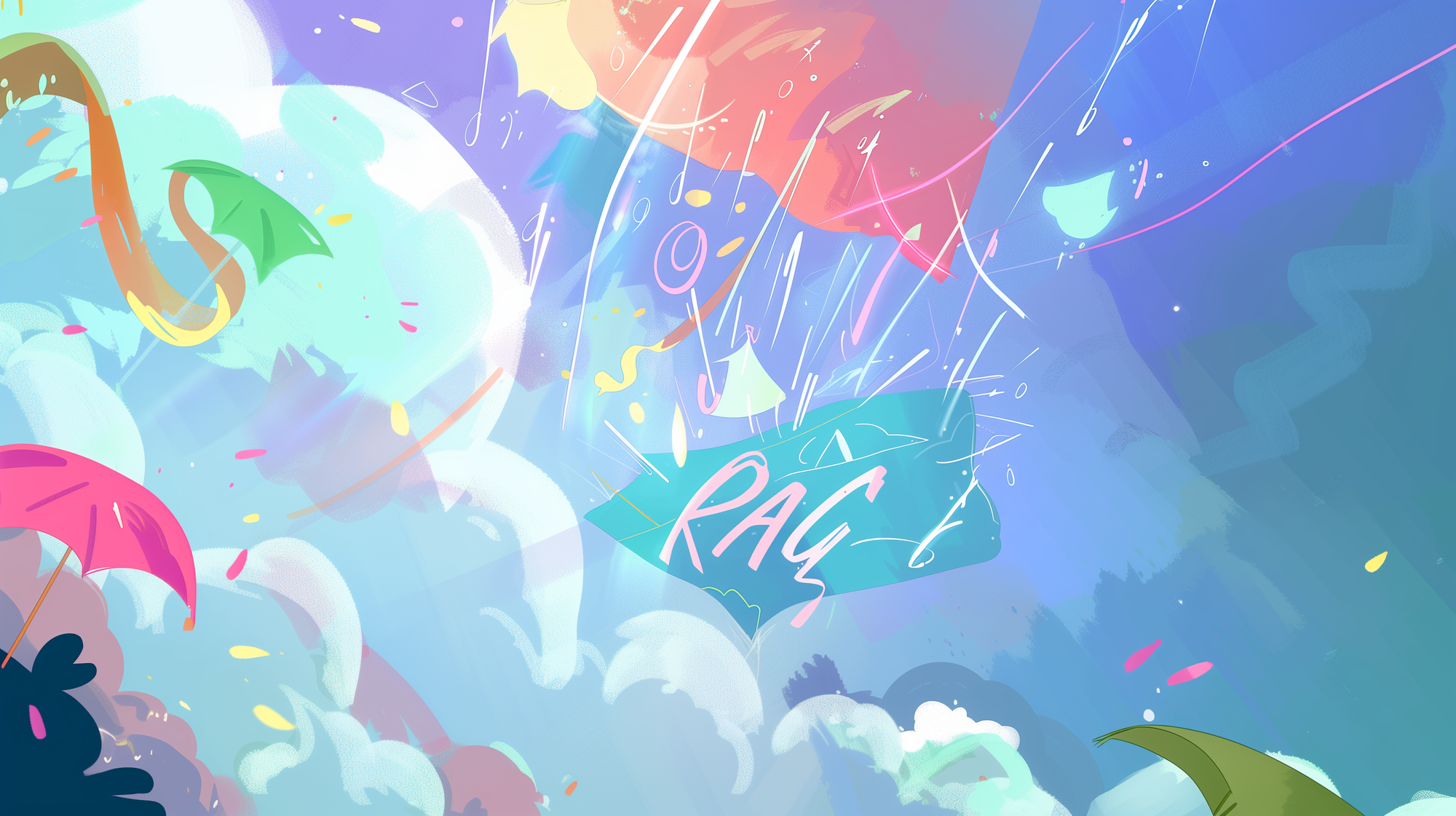
Matthew Berman breaks down the transformative power of RAG and its potential to redefine large language models.
Understanding the nuances of technologies that drive progress is more than a matter of academic interest—it's essential for anyone looking to harness AI's full potential. Among these technologies, Retrieval Augmented Generation (RAG) emerges as a key player, frequently overshadowed by more common approaches like fine-tuning, yet offering unique advantages that could redefine the capabilities of large language models. But what is RAG, and how does it work? 🤔
At its core, RAG is about enhancing AI's ability to process and utilise information, bridging the gap between the vast knowledge already existing in various databases and the AI's need to access this knowledge efficiently. This breakthrough addresses a fundamental limitation of large language models: their static nature upon training completion. Imagine an AI that can't learn about the latest scientific discoveries or current events because it stopped updating the moment its training ended. That's where Rag comes into play!
But RAG's implications extend far beyond just keeping AI informed. Its ability to tap into external knowledge sources and append only the most relevant data to prompts saves precious context window space, allowing models to operate more intelligently and with greater relevance. Moreover, by integrating embedding models and vector databases, RAG paves the way for incredibly responsive AI systems capable of sophisticated, multi-step problem-solving. And companies like Pine Cone are making these advanced technologies more accessible than ever, requiring less technical knowledge to implement effectively.
Reflecting on RAG's Potential
In a world where customer service chatbots understand your query in a nuanced way, providing not just any response, but the most accurate, up-to-date information available. Or consider the power of querying company documents with natural language, receiving precise answers in milliseconds. This is the world RAG is helping to create.
Yet, with all these advancements, questions about RAG's application, efficiency, and future potential naturally arise. How do these technologies fit within our broader AI strategies? What challenges do we face in integrating RAG into existing models?
YOUR HOMEWORK:
Jump into Perplexity & Ask Questions
- What are the main functions and advantages of Retrieval Augmented Generation (RAG) for artificial intelligence?
- In what ways does fine-tuning large language models differ from the benefits provided by RAG?
- How does the "frozen in time" nature of large language models without RAG limit their effectiveness?
- Why is the context window limitation significant in large language models, and how does RAG address it?
- Can you explain the role of embedding models in the context of RAG and language processing?
- What is the significance of vector databases in the efficiency of RAG implementations?
- How does RAG prevent hallucination in responses produced by large language models?
- How might customer service chatbots benefit from being augmented with RAG?
- What are some examples of sophisticated, multi-step problem-solving that could be enabled by RAG?
- Why might Pine Cone's vector storage solution be deemed pivotal for RAG?
- How can RAG contribute to the continuous updating of information for large language models?
- What implications do the larger context windows of LAMA 3 and GPT-4 have for efficiency and cost?
- How do embedding models and vector databases contribute to creating highly responsive AI systems?
- Can you discuss how RAG allows for integration of multiple external knowledge sources iteratively?
- What makes Pine Cone user-friendly for those looking to implement RAG without extensive technical knowledge?